How AI Can Improve Your Accounts Receivable Process: The Future Of AR Automation
January 9, 2025How AI Can Improve Credit Risk Management: A Smarter Way to Protect Your Receivables
April 24, 2025Introduction
The evolution of technology has dramatically reshaped the way businesses operate. From standalone applications to cloud-based SaaS solutions and now AI-powered platforms, each phase has brought about increased efficiency and scalability. In the realm of finance, particularly Accounts Receivable (AR) management, these advancements have redefined processes that were once manual and time-consuming.
AI is not just an option but a necessity for businesses aiming to optimize their AR processes. By leveraging AI-driven insights, automation, and decision-making capabilities, companies can mitigate the inefficiencies of traditional AR management and improve cash flow predictability.
The Shortcomings of the Manual Accounts Receivable Process
Traditional AR management involves tracking invoices, following up on payments, and reconciling accounts. While these tasks are essential, manual execution poses several challenges:
- Human Errors: Manual data entry increases the risk of mistakes, leading to incorrect invoices, missed payments, and reconciliation issues.
- Delays in Collections: Dependence on spreadsheets and ERP systems with limited automation capabilities often results in inefficiencies, slowing down the collections process.
- Limited Visibility: Without real-time insights, businesses struggle to forecast cash flow accurately, impacting financial planning and decision-making.
The Impact of Traditional AR Processes on Business Performance
Relying solely on ERP systems and spreadsheets for AR management can lead to:
- Increased Days Sales Outstanding (DSO), reducing available working capital.
- Inefficient cash flow planning due to inaccurate forecasting.
- High operational costs due to manual follow-ups and dispute resolution.
Scenarios That Slow Down Collections and Affect AR Performance
- Delayed Follow-Ups: A collection specialist manually following up with customers may miss crucial payment deadlines due to workload constraints.
- Dispute Resolution Bottlenecks: A company manually reviewing disputes takes weeks to resolve an issue, delaying payments and affecting cash flow.
- Lack of Payment Insights: Without AI-driven analytics, businesses fail to anticipate potential late payments, resulting in unplanned cash shortages.
Transitioning to AI-Powered AR Management Systems
AI-driven Accounts Receivable management systems are designed to address inefficiencies through automation, predictive analytics, and real-time decision-making. These intelligent systems improve financial operations by reducing manual tasks, accelerating cash flow, and minimizing risks associated with late payments and bad debt.
Context-Aware AI-Powered Accounts Receivable Systems
These systems use machine learning models to understand customer behavior, payment patterns, and business rules to support finance teams in:
- Automating Invoice Processing: AI automates the generation, validation, and tracking of invoices, ensuring timely payments.
- Enhancing Payment Predictions: AI models analyze past payment behavior to predict future payment trends, allowing businesses to take preemptive action.
- Streamlining Communication with Customers: AI-powered chatbots and automated emails personalize and optimize payment follow-ups, improving response rates.
- Providing Proactive Dispute Resolution: AI can identify common dispute patterns, categorize claims, and suggest resolutions before they escalate.

What Are Accounts Receivable AI Agents?
AI agents in Accounts Receivable management act as virtual assistants that can:
- Detect potential payment delays and recommend proactive actions to avoid cash flow disruptions.
- Automate follow-ups with customers based on payment history and personalized communication strategies.
- Categorize and prioritize disputes for faster resolution, reducing operational burden on finance teams.
Accounts Receivable AI agents have emerged as a transformative solution, embedding industry-specific expertise into real-time, adaptable AR systems. By combining the flexibility of context-aware systems with domain-specific knowledge, these AI-driven agents empower organizations to tackle AR challenges with precision and efficiency.
Operational Advantages of Accounts Receivable AI Agents

1. Targeted Domain Expertise
Accounts Receivable AI agents are fine-tuned to handle the complexities of AR processes in an industry ensuring:
- Automated contract analysis to verify payment terms and flag discrepancies before invoicing.
- Risk assessment for outstanding receivables, using historical payment behavior to predict late payments and suggest proactive actions.
- Compliance with financial regulations through AI-driven checks that ensure adherence to accounting standards and policies.
2. Dynamic Adaptability in Real-Time Operations
Unlike rigid legacy systems, AI-driven AR solutions adjust dynamically to payment trends and external factors. This includes:
- Real-Time Payment Monitoring: AI agents detect irregular payment patterns, alerting teams to potential fraud or delayed payments.
- Automated Payment Prioritization: By analyzing due dates and risk levels, AI recommends collection strategies that optimize recovery rates.
- Adaptive Workflows: AI dynamically updates AR processes based on customer interactions, seasonal trends, or macroeconomic conditions.
3. End-to-End Workflow Automation
By automating complex AR workflows, Accounts Receivable AI agents transform raw financial data into actionable insights. Benefits include:
- Faster Invoice Processing: AI enables instant invoice validation, reducing processing delays.
- Reduced Operational Costs: Automating repetitive tasks such as reconciliation allows finance teams to focus on strategic decisions.
- Seamless ERP Integration: AI bridges gaps between structured (e.g., ERP systems) and unstructured data (e.g., emails, contracts), ensuring smooth financial operations
Understanding Accounts Receivable LLM Agents
Large Language Model (LLM) agents are advanced AI models designed to handle complex AR-related interactions. They assist in:
- Generating contextual responses for customer queries.
- Summarizing past interactions to provide personalized follow-ups.
- Enhancing AR workflows by automating decision-making.
LLM Agents vs. LLM Workflows in AR
While both are AI-driven, they serve different purposes:
- LLM Agents operate independently, handling real-time customer interactions and decision-making.
- LLM Workflows orchestrate multiple AI agents and automation steps to streamline end-to-end AR processes.
Core Modules of Accounts Receivable LLM Agents

1. Memory
The memory module plays a crucial role in maintaining the continuity and personalization of interactions. Key functions include:
- Retaining Customer Payment History and Preferences: This module securely stores detailed records of each customer’s payment history, including past due amounts, payment schedules, and preferred payment methods.
- Enhancing Personalized Follow-Ups: By leveraging this historical data, the system can provide more personalized follow-up actions, such as reminders tailored to the customer’s payment behavior, thus improving collection efficiency and customer satisfaction.
2. Reasoning Engine
The reasoning engine is the analytical brain of the system, designed to assess and make recommendations based on data insights. Key functions include:
- Assessing Payment Patterns and Recommending Actions: It analyzes the payment behavior of customers to identify patterns, such as regular late payments or partial payments. Based on these patterns, it recommends appropriate actions, like sending reminders or offering payment plans.
- Prioritizing Collections Efforts: By evaluating the risk and likelihood of payment from different customers, it helps prioritize efforts, focusing on high-risk accounts that need immediate attention while automating routine follow-ups for low-risk accounts.
3. Cognitive Skills – Task-Specific Inferences
This module leverages advanced AI to handle specific, complex tasks that require a higher level of cognitive processing. Key functions include:
- AI-Driven Dispute Categorization and Risk Assessment: When a payment dispute arises, the system can categorize the dispute type and assess the associated risk. This allows for faster resolution and better risk management.
- Predictive Analytics for DSO Reduction: Using predictive analytics, it forecasts the days sales outstanding (DSO) and suggests strategies to reduce it. This might involve identifying potential payment delays before they occur and taking preemptive action.
By integrating these core modules, Accounts Receivable LLM Agents can significantly enhance the efficiency, accuracy, and effectiveness of accounts receivable management, ultimately improving cash flow and customer relations.
Agentic Systems in AR Management
Characteristics of AI Agentic Systems for Accounts Receivable (AR)
Autonomous Decision-Making: AI systems are equipped to evaluate accounts receivable (AR) data and make decisions without human intervention. They can execute predefined actions such as sending payment reminders, initiating follow-ups, and offering payment plans based on the analysis of customer payment behavior.
Context Awareness: These systems understand the historical context of each customer interaction. They adapt communication strategies based on the customer’s payment history, preferences, and prior engagements, leading to more personalized and effective interactions.
Continuous Learning: AI agentic systems continuously learn from historical data and past interactions. They refine their strategies over time, enhancing their accuracy and effectiveness in predicting payment patterns, categorizing disputes, and recommending actions.
Types of AI Agents in Accounts Receivable
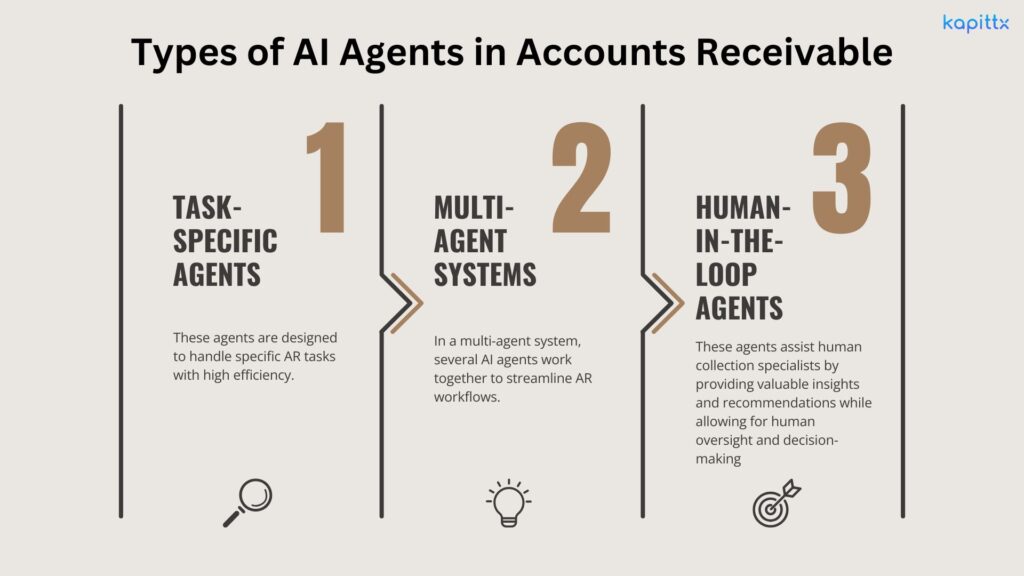
1. Task-Specific Agents: These agents are designed to handle specific AR tasks with high efficiency. For example, they can manage invoice tracking, ensuring all invoices are accounted for and followed up promptly. They also handle dispute resolution by categorizing disputes, assessing risks, and suggesting solutions based on historical data.
2. Multi-Agent Systems: In a multi-agent system, several AI agents work together to streamline AR workflows. Each agent may be responsible for a different aspect of the AR process, such as invoicing, payment reminders, dispute management, and risk assessment. By collaborating, these agents can provide a comprehensive AR management solution that enhances overall efficiency and effectiveness.
3. Human-in-the-Loop Agents: These agents assist human collection specialists by providing valuable insights and recommendations while allowing for human oversight and decision-making. They analyze data and suggest actions, but the final decisions are made by human experts, combining the strengths of AI and human intuition.
The Future of AI in Accounts Receivable
AI-Driven Negotiation and Dispute Resolution: As AI technology continues to evolve, AI-powered assistants will become more adept at handling negotiations and resolving disputes. They will facilitate smoother dispute resolution processes, reducing the time required to settle issues and improving overall customer satisfaction.
Intelligent Credit Risk Assessment: Future AI agents will have the capability to analyze real-time financial data, providing more accurate and timely assessments of customer creditworthiness. This will enable businesses to make informed decisions about extending payment terms and managing credit risk more effectively.
Advanced Predictive Analytics: AI-powered dashboards will offer CFOs and finance teams actionable insights for optimizing working capital. These dashboards will leverage predictive analytics to forecast cash flow, identify potential payment delays, and suggest strategies for improving AR management and reducing days sales outstanding (DSO).
By integrating these advanced AI capabilities, AR management systems will become more efficient, accurate, and proactive, ultimately enhancing the financial health of businesses and their relationships with customers.
Conclusion and Future Directions
AI is revolutionizing accounts receivable management by enhancing efficiency, accuracy, and customer experiences. AI Agents and LLM-powered automation platforms like Kapittx are leading this transformation by providing businesses with intelligent, automated solutions that drive faster payments and optimize cash flow.
The transformation of AR management through AI is not just an upgrade—it is a strategic necessity. Businesses that adopt AI-powered AR solutions gain a competitive edge by improving efficiency, reducing DSO, and optimizing cash flow.
Kapittx is at the forefront of this revolution, offering AI-driven solutions tailored to modern financial needs. Now is the time to define your AI roadmap with Kapittx and unlock the true potential of intelligent AR management.
FAQ
How to Use AI to Improve Accounts Receivable?
AI can significantly enhance Accounts Receivable (AR) management by automating tasks, improving accuracy, and providing valuable insights. Here’s how:
Enhanced Reporting: AI-powered dashboards provide real-time insights and detailed reports, aiding decision-making.
Automated Payment Reminders: AI can automatically send payment reminders based on customer behavior and payment history.
Predictive Analytics: AI can forecast payment delays, assess credit risk, and suggest strategies to reduce Days Sales Outstanding (DSO).
Dispute Resolution: AI can categorize and assess disputes, recommending solutions based on historical data.
Customer Segmentation: AI can segment customers based on payment patterns, allowing for tailored communication strategies.
What Are Accounts Receivable AI Agents?
Accounts Receivable AI Agents are intelligent systems designed to manage and optimize the AR process. They use machine learning and AI algorithms to automate tasks, analyze data, and provide actionable insights, improving efficiency and reducing manual efforts.
What Are Accounts Receivable LLM Agents?
Accounts Receivable Large Language Model (LLM) Agents leverage advanced language models to enhance AR management. These agents can understand and process natural language, enabling them to communicate effectively with customers, resolve disputes, and generate insights from unstructured data.
What Are the Types of AI Agents in Accounts Receivable?
Task-Specific Agents: Focused on specific AR tasks like invoice tracking, dispute resolution, and payment reminders.
Multi-Agent Systems: Multiple AI agents collaborating to streamline AR workflows, each handling different aspects of the AR process.
Human-in-the-Loop Agents: AI assists human specialists by providing insights and recommendations, with final decisions made by humans.
What Are Agentic Systems in Accounts Receivable Management?
Agentic Systems in AR management refer to AI-driven systems that exhibit characteristics such as:
- Autonomous Decision-Making: Evaluating AR data and taking predefined actions without human intervention.
- Context Awareness: Understanding customer history and adapting communication accordingly.
Continuous Learning: Refining strategies based on historical data to improve accuracy and effectiveness.
What Are the Advantages of Accounts Receivable AI Agents?
Efficiency: Automation of repetitive tasks reduces manual effort and speeds up processes.
Accuracy: AI minimizes human errors, ensuring accurate data processing and analysis.
Personalization: AI tailors communication based on customer behavior and history, improving customer relationships.
Predictive Insights: AI provides predictive analytics to forecast payment delays and suggest proactive measures.
Cost Savings: Reduced manual work and improved efficiency lead to cost savings.
What Are the Core Modules of Accounts Receivable LLM Agents?
Memory: Retains customer payment history and preferences, enhancing personalized follow-ups.
Reasoning Engine: Assesses payment patterns and recommends actions, helping prioritize collections efforts.
Cognitive Skills – Task-Specific Inferences: AI-driven dispute categorization and risk assessment, predictive analytics for DSO reduction.
What Are Context-Aware Accounts Receivable Systems?
Context-Aware AR Systems are AI-driven systems that understand and respond to the context of customer interactions. They leverage customer history, payment behavior, and communication preferences to adapt their strategies and provide personalized solutions. These systems enhance customer experience and improve AR management by ensuring relevant and timely communication.
What are the challenges of using AI in Accounts Receivable?
Using AI in Accounts Receivable (AR) management can offer numerous benefits, but it also comes with its own set of challenges. Here are some key challenges:
Data Quality and Integration
- Inconsistent Data: AI systems require high-quality, consistent data to function effectively. Inaccurate or incomplete data can lead to incorrect predictions and recommendations.
- Integration with Existing Systems: Integrating AI with existing ERP and AR systems can be complex and time-consuming. Ensuring seamless data flow between systems is crucial for effective AI implementation.
Change Management
- Employee Resistance: Employees may resist adopting AI-driven solutions due to fear of job displacement or reluctance to change established processes.
- Training and Adaptation: Effective implementation requires training employees to work with AI systems and adapting existing workflows to incorporate AI insights.
Algorithm Bias
- Bias in AI Models: AI models can inherit biases present in the training data, leading to unfair or skewed recommendations. It’s important to regularly audit and update AI models to mitigate bias.
Cost and Resources
- Initial Investment: Implementing AI solutions can involve significant initial investment in software, hardware, and integration services.
- Ongoing Maintenance: AI systems require continuous monitoring, maintenance, and updates to ensure they remain effective and accurate.
Data Security and Privacy
- Sensitive Information: AR management involves handling sensitive financial data. Ensuring data security and compliance with privacy regulations is critical.
- Cybersecurity Risks: AI systems can be targets for cyberattacks, making robust cybersecurity measures essential.
Predictive Accuracy
- Uncertainty in Predictions: While AI can provide valuable insights, its predictions are not always accurate. External factors and unforeseen events can affect payment behaviors.
- Dependence on Historical Data: AI predictions are based on historical data, which may not always reflect future trends and behaviors accurately.
Regulatory Compliance
- Compliance Challenges: Ensuring that AI systems comply with financial regulations and industry standards is crucial to avoid legal issues.
- Keeping Up with Regulations: Financial regulations are continuously evolving, and AI systems must be regularly updated to remain compliant.
While these challenges exist, they can be managed with careful planning, continuous monitoring, and a proactive approach to addressing potential issues. The benefits of using AI in AR management often outweigh the challenges, leading to improved efficiency, accuracy, and decision-making in the long run.